Options
A New Motion Segmentation Technique using Foreground-Background Bimodal
Journal
Malaysian Journal of Science, Health & Technology
Date Issued
2018
Author(s)
Al-Smadi, M.
Abdul Rahim, K.
Abdul Salam, R.
DOI
10.33102/mjosht.v2i.44
Abstract
Vehicle detection is a fundamental step in urban traffic surveillance systems, since it provides necessary information for further processing. Conventional techniques utilize either background subtraction or foreground appearance-based detection, which involves either poor adaptation or high computation. The complexity of urban traffic scenarios lies in pose and orientation variations, slow or temporarily stopped vehicles and sudden illumination variations. In this work, a foreground-background bimodal is proposed to adapt for scene variation and complexity. Cumulative frame differencing and sigma-delta estimation are used to model foreground and background respectively. A correction feedback updates each model iteratively and recursively based on the detection mask of the other model. Variance update for sigma-delta estimation was limited to update background temporal activities, while cumulative frame differencing account for moving foreground by discarding limited background variations. Comparative experimental results for typical urban traffic sequences show that the proposed technique achieves robust and accurate detection, which improves adaptation, reduce false detection and satisfy real-time requirements.
File(s)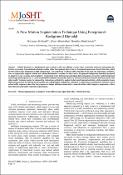
Loading...
Name
A New Motion Segmentation Technique Using ForegroundBackground Bimodal.pdf
Size
875.36 KB
Format
Adobe PDF
Checksum
(MD5):4b450277aba8c32ff19fd6e2d3da1e84